
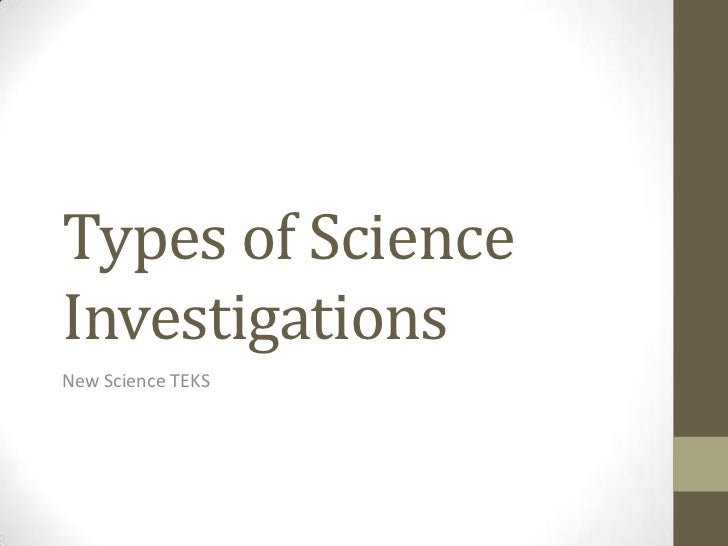
By utilizing the optical transfer function (OTF) of certain imaging modalities and the spatiotemporal continuity of noisy image series, the researchers constructed a learnable Fourier noise suppression module (FNSM) and the temporally/spatially interleaved self-supervised rDL (TiS/SiS-rDL) denoising networks, which can be implemented without the need to acquire ground-truth (GT) SR training data, but still yield results as good, sometimes even better, as the ones if supervised with GT, therefore, eliminating the need for extra training data. The rDL concept is of wide compatibility and broad functionality. Systematic comparison demonstrated that the physical model rationalized three-branch architecture significantly improves the SR information by >10-fold and alleviates the model-uncertainty by 3~5-fold, providing physically feasible inferences that can be more readily generalized onto the regimes uncovered by training data. This strategy eliminates the spectral-bias effect of recovering Moiré fringes, but also ensures the high-quality SR image reconstruction without spatial and temporal resolution degradation.
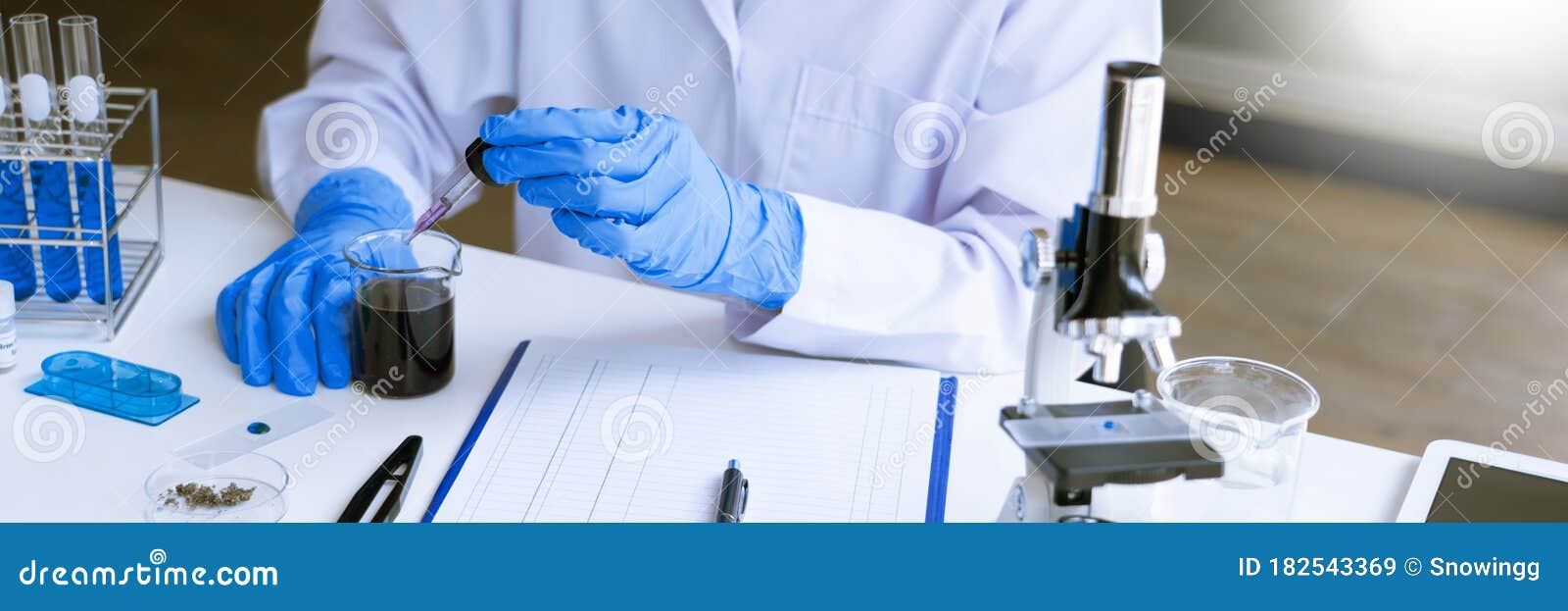
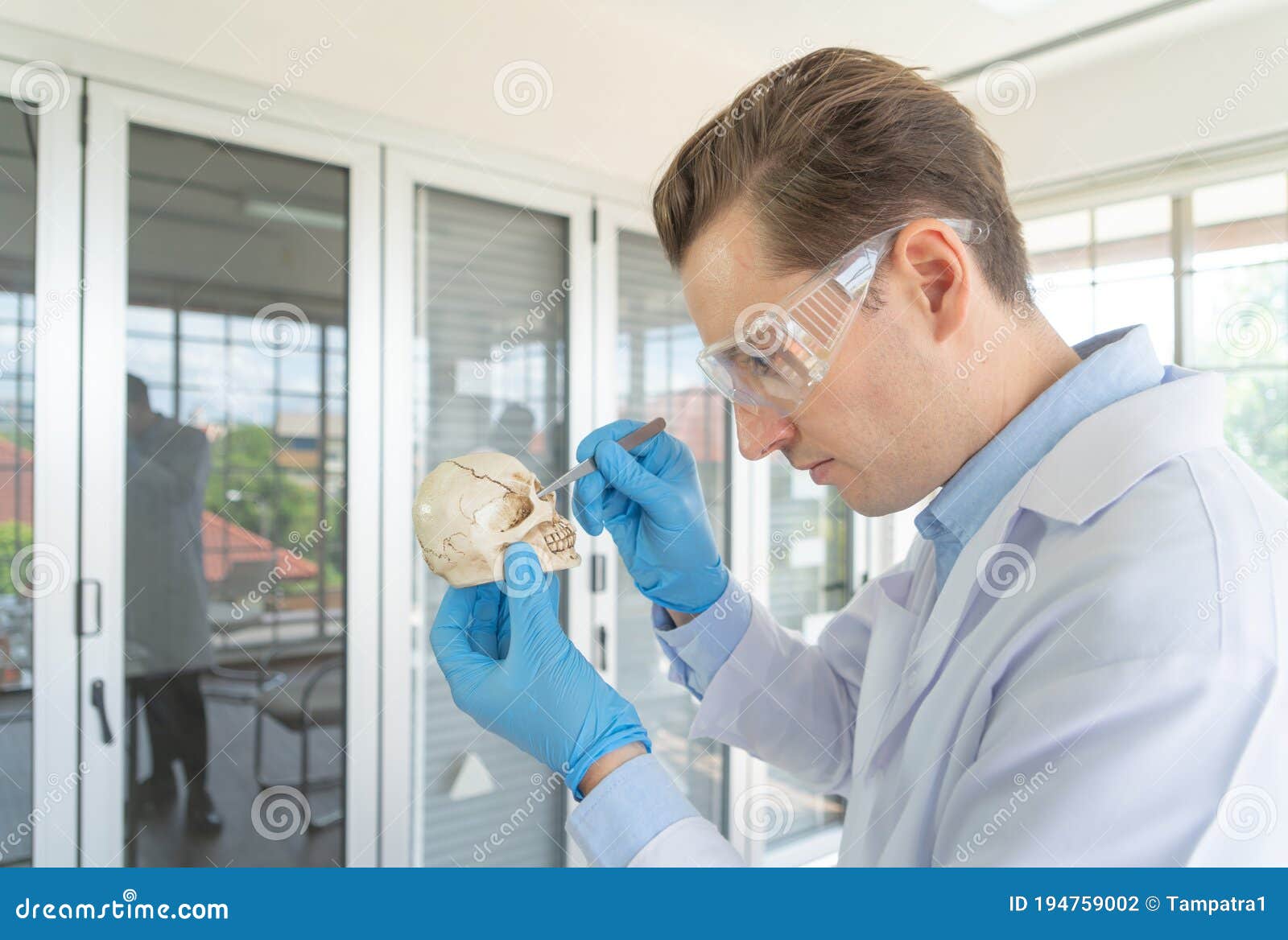
Instead of directly inferring the SR images as current super-resolution neural networks trained in an end-to-end manner, the rDL methods are applied to denoise the raw images, in which the high-frequency information beyond the diffraction limit is down-modulated as low-frequency Moiré fringes, and then reconstruct the SR image using the well-established SIM algorithm.
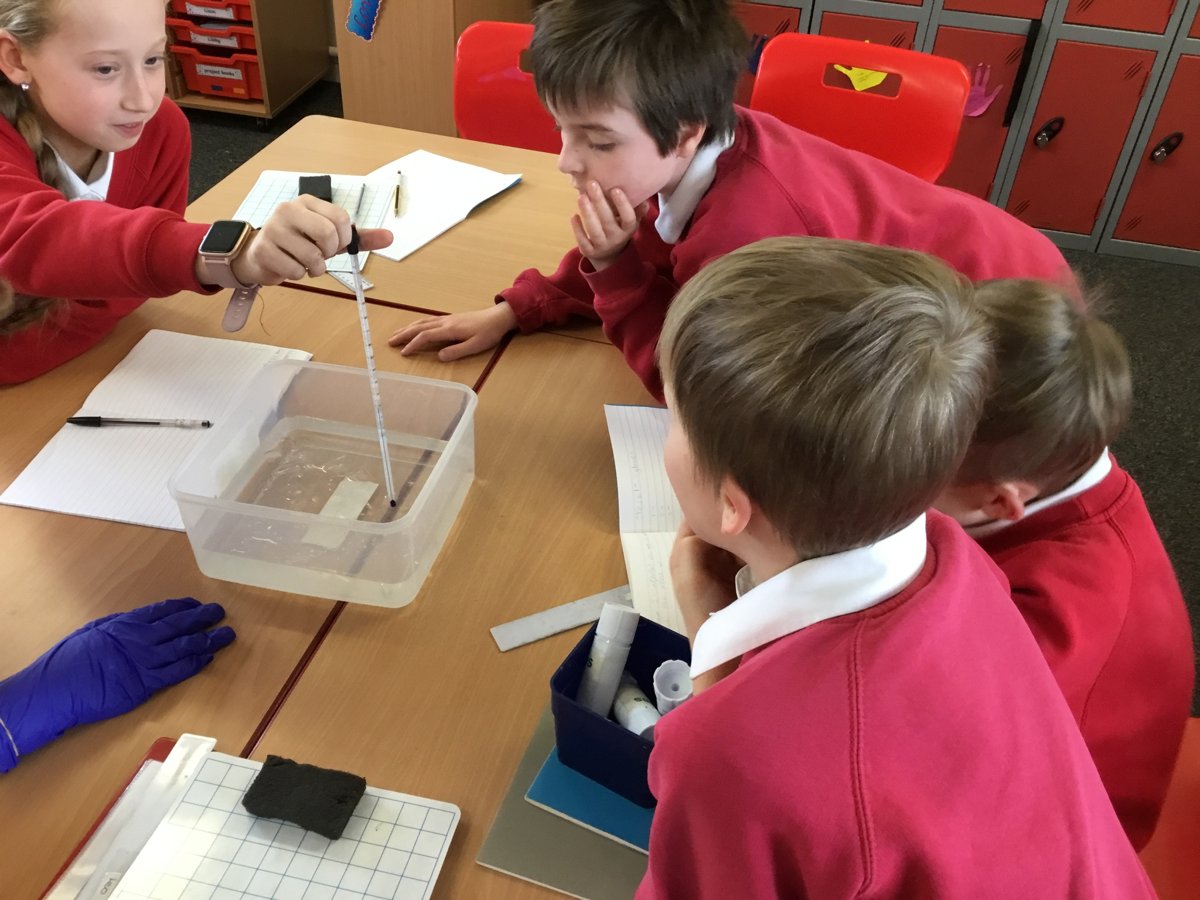
They demonstrated that the rDL-SRM offers the first practical solutions for imaging a variety of dynamic intracellular processes at ultrahigh resolution in space and time for an unprecedented duration, including rapid kinetics inside motile cilium, the multi-phase transition of nucleolar proteins over light-sensitive mitosis, and long-time interactions between membranous and membrane-less organelles. The researchers reported a method of rationalized deep learning super-resolution microscopy (rDL-SRM), which implants a deterministic physical model of specific microscopy into the network training and inference to eliminate/minimize the ill-posedness, model-uncertainty, spectral-bias, and demands for high-quality training dataset in current deep learning super-resolution (DLSR) methods.
